Uncertainty Quantification (UQ) Group at Duke University
We develop methods, models and algorithms at the intersection of Computational Mechanics, Materials Science, (Probability Theory and Statistics) ⊃ Uncertainty Quantification, and Scientific Machine Learning. We combine physics-based constraints with mathematical representations to enhance predictive capabilities in a variety of challenging problems across a wide span of scales, ranging from atomistic simulations on next-generation batteries to continuum models for additive manufacturing, biomedical implants, and naval applications (to name a few). We have tested our approaches in collaborative projects with Sandia National Laboratories and the U.S. Naval Research Laboratory.
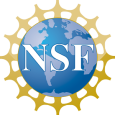
Financial support from the Army Research Office, Duke University, the National Science Foundation, the U.S. Naval Research Laboratory, and Sandia National Laboratories is gratefully acknowledged.